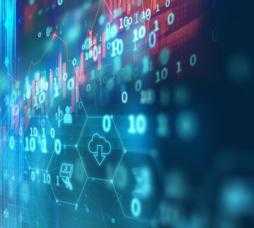
Faced with this problem, many researchers and engineers, in both public research and industry, are developing approaches, methods and tools to better document and reduce the environmental impacts associated with machine learning models. Numerous avenues of optimization are being explored, such as software optimization and frequent hardware changes to take advantage of maximum energy efficiency. Or even relocating computations to areas with less carbon-intensive electricity. All this optimization work must not overlook two crucial factors: the displacement of impacts, and the growth trends in digital and AI that these optimizations help to continue.
What's more, even if the environmental issues associated with the production and use of machine learning models were to be resolved by these methods, many other issues, notably ethical and social, would persist. The aim of this seminar is to provide an overview of the environmental and social impacts of AI, and the strategies being devised to address them.